Uncovering The Hidden Secrets of Semantic Search
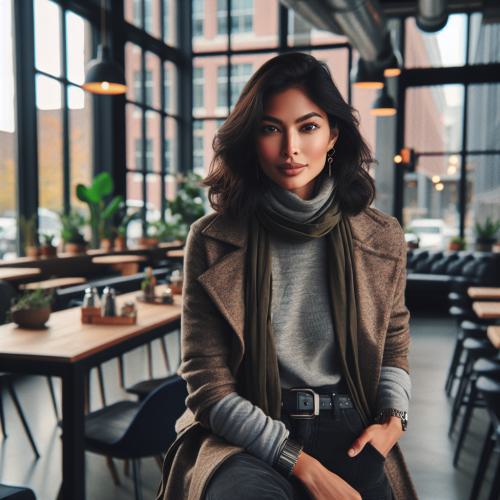
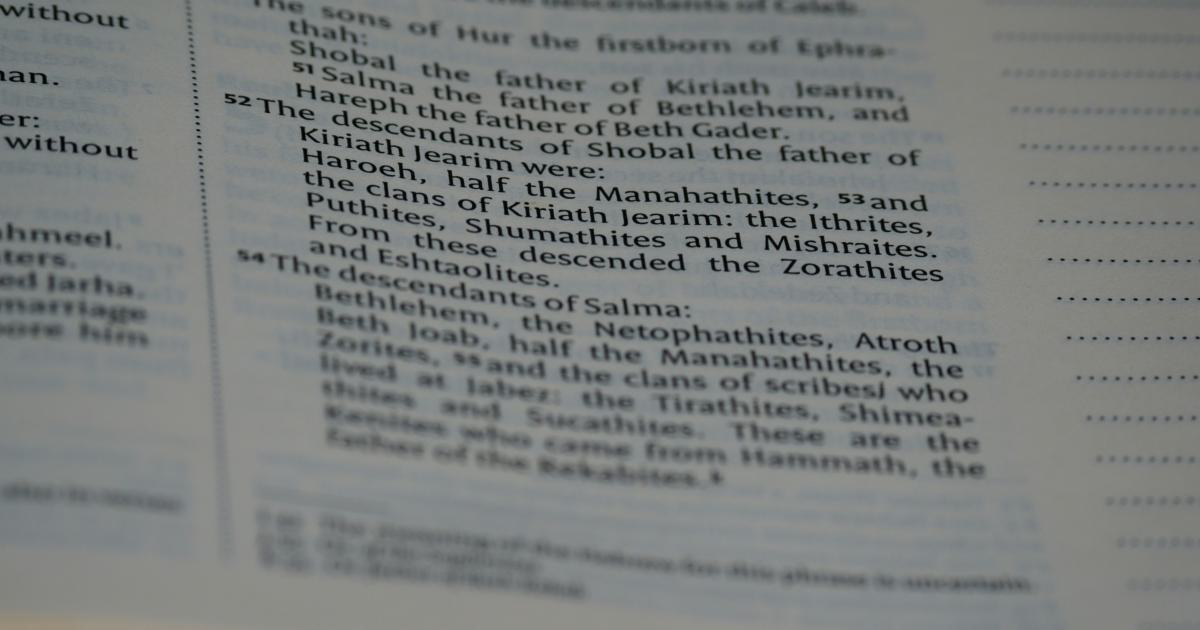
Introduction to Semantic Search
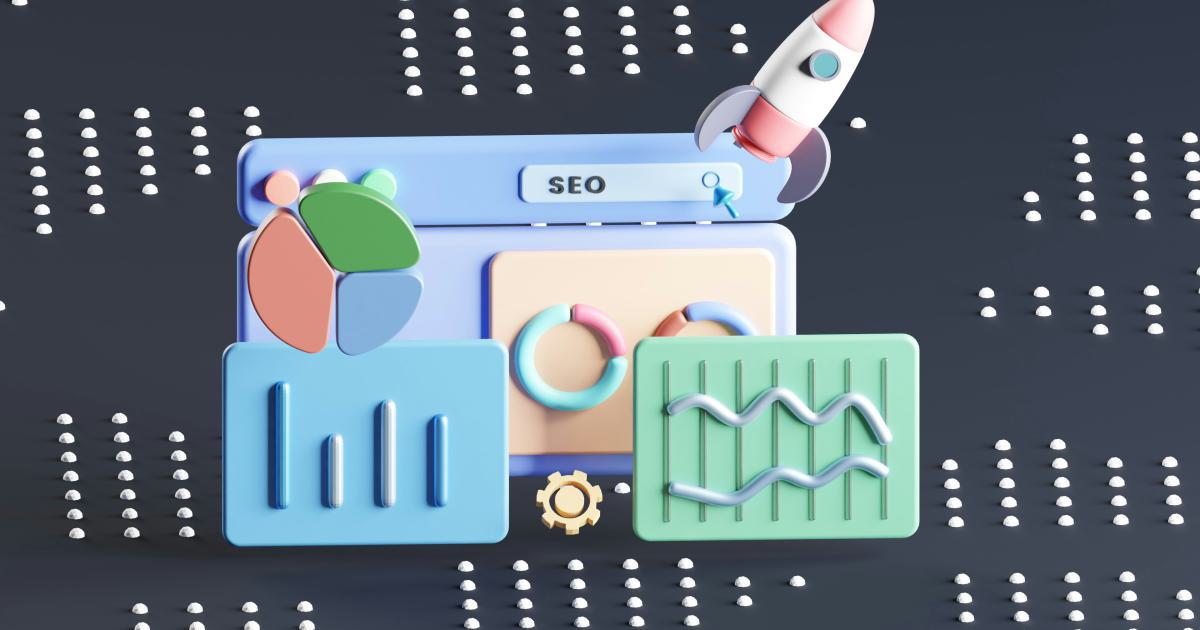
In the ever-evolving world of digital information, the way we search for and retrieve relevant content has undergone a significant transformation. Traditional keyword-based search has long been the dominant method, but as the volume and complexity of online data continue to grow, the need for a more sophisticated approach has become increasingly apparent. Enter semantic search, a powerful technology that promises to revolutionize the way we interact with and make sense of digital information.
Semantic search is a search technique that goes beyond the literal interpretation of search queries, aiming to understand the underlying meaning and intent behind the user's request. Unlike traditional keyword-based search, which relies on a surface-level matching of terms, semantic search utilizes advanced natural language processing (NLP) and machine learning algorithms to grasp the contextual significance of the search query, taking into account factors such as synonyms, related concepts, and user intent.
By delving deeper into the semantics of the search query, semantic search engines can provide users with more accurate, relevant, and personalized results, ultimately enhancing the overall search experience. This shift in approach has the potential to unlock a wealth of hidden insights and uncover new avenues for discovery, transforming the way we access and leverage digital information.
The Evolution of Search: From Keyword to Semantic
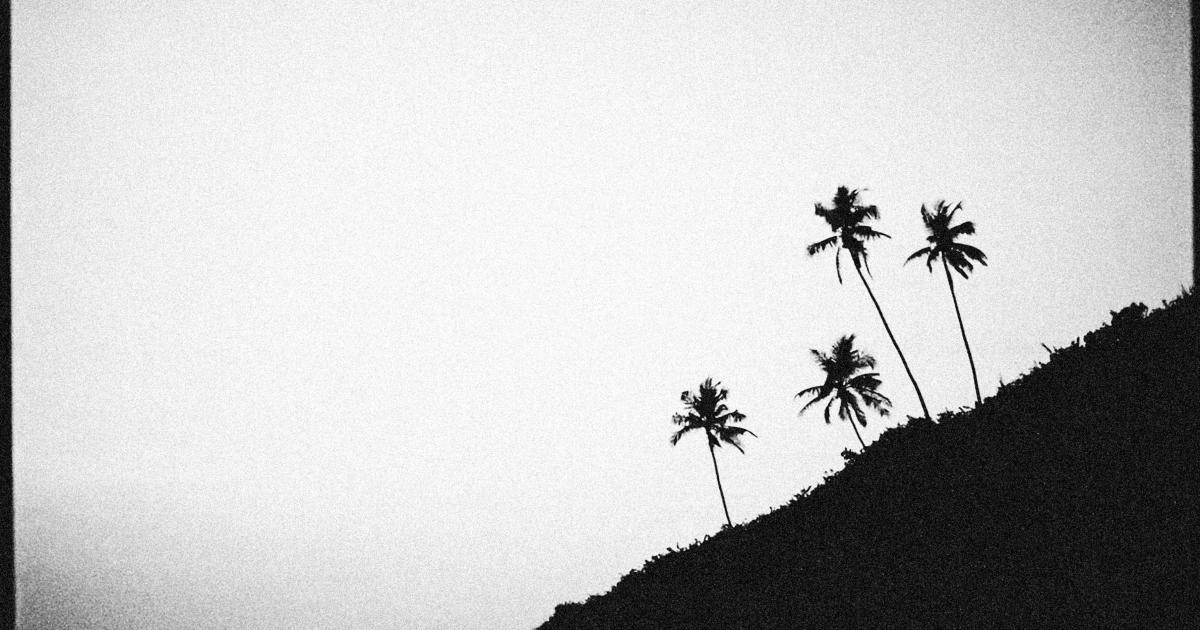
The progression from traditional keyword-based search to semantic search has been a gradual, yet transformative journey. In the early days of the internet, search engines relied heavily on the literal matching of keywords, often resulting in a deluge of irrelevant or imprecise results.
As the volume of online content grew exponentially, the limitations of keyword-based search became increasingly apparent. Users found themselves sifting through pages of results, often struggling to find the information they were truly seeking. This realization paved the way for the emergence of semantic search, a more sophisticated approach that aimed to understand the underlying meaning and context of search queries.
The key drivers behind the evolution of search include:
Exponential Growth of Online Data: The sheer volume and diversity of digital content have rendered traditional keyword-based search increasingly ineffective, as it struggles to keep up with the ever-expanding information landscape.
Changing User Behavior and Expectations: Modern users expect more personalized, contextual, and intuitive search experiences, moving beyond the simple matching of keywords to a more nuanced understanding of their needs and intent.
Advancements in Natural Language Processing (NLP): Significant strides in NLP, machine learning, and artificial intelligence have enabled search engines to better comprehend the complexities of human language, unlocking the potential for more sophisticated semantic search capabilities.
The Rise of Voice Search and Virtual Assistants: The increasing popularity of voice-based search and virtual assistants has further driven the need for search engines to understand natural language and user intent, as these technologies rely heavily on contextual understanding.
As search engines continue to evolve, the incorporation of semantic search principles has become a crucial step in delivering more accurate, relevant, and personalized search results. By uncovering the hidden meanings and relationships within search queries, semantic search promises to elevate the overall user experience and unlock new opportunities for discovery and knowledge sharing.
The Principles of Semantic Search
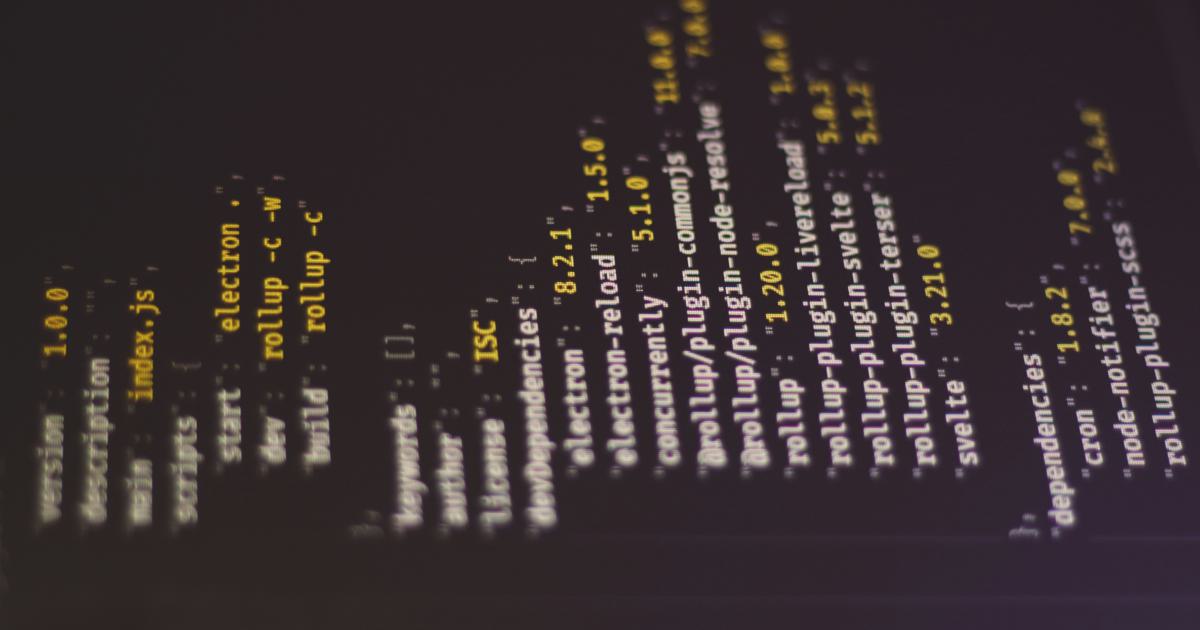
At the heart of semantic search lies a deep understanding of the underlying meaning and context of search queries. Instead of simply matching keywords, semantic search engines utilize a range of advanced techniques to uncover the true intent behind a user's search, ultimately delivering more relevant and valuable results.
Contextual Understanding
One of the core principles of semantic search is its ability to analyze the context surrounding a search query. This includes factors such as the user's search history, location, device, and even the time of day. By considering these contextual clues, semantic search engines can better interpret the user's intent and provide results that are tailored to their specific needs and preferences.
For example, if a user searches for "best Italian restaurant," a semantic search engine would not only look for web pages mentioning "Italian restaurant," but also consider the user's location, past search history, and other contextual factors to determine the most relevant and personalized results.
Conceptual Matching
Traditional keyword-based search relies on the literal matching of terms, which can often fall short in capturing the broader meaning and relationships between search queries and content. Semantic search, on the other hand, employs conceptual matching, which involves understanding the underlying concepts and ideas behind the search query.
This approach allows semantic search engines to recognize synonyms, related terms, and even infer user intent beyond the literal search terms. For instance, a search for "automobile" may also surface results related to "car," "vehicle," or "transportation," as the semantic search engine recognizes the conceptual connections between these terms.
Entity Extraction and Mapping
Semantic search engines often utilize entity extraction and mapping techniques to enhance their understanding of search queries. By identifying and analyzing the entities (such as people, places, organizations, or events) mentioned within a search query, these engines can better comprehend the context and meaning of the user's request.
For example, if a user searches for "Barack Obama," a semantic search engine would recognize "Barack Obama" as a person and provide results related to the former U.S. president, his biography, policies, and other relevant information, rather than simply returning web pages that mention the name "Barack Obama" without any contextual understanding.
Knowledge Graph Integration
Many leading search engines, such as Google, have incorporated knowledge graphs into their semantic search algorithms. A knowledge graph is a structured database that stores information about entities, their properties, and the relationships between them. By integrating knowledge graphs, semantic search engines can better understand the broader context and conceptual connections within search queries, enabling more informed and insightful results.
For instance, a search for "Eiffel Tower" would not only return information about the iconic Parisian landmark, but also related facts, such as its location, construction history, and cultural significance, all made possible by the search engine's access to a comprehensive knowledge graph.
Natural Language Processing (NLP)
At the core of semantic search lies the application of advanced natural language processing (NLP) techniques. NLP algorithms are designed to analyze and comprehend the nuances of human language, including grammar, syntax, and the intended meaning behind search queries.
By leveraging NLP, semantic search engines can better interpret the user's intent, even in the case of complex, ambiguous, or conversational search queries. This allows for a more natural and intuitive search experience, where the search engine can understand the broader context and provide more relevant and helpful results.
The Benefits of Semantic Search
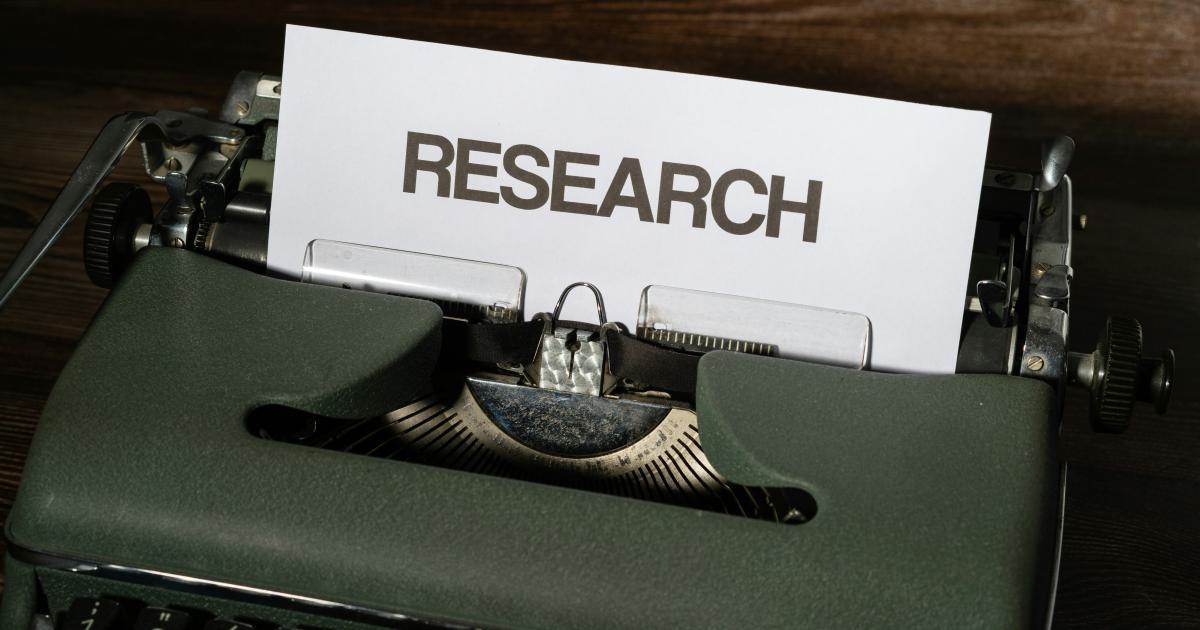
The adoption of semantic search technology offers a multitude of benefits for both users and businesses, transforming the way we interact with and leverage digital information.
Improved Relevance and Accuracy
One of the primary advantages of semantic search is its ability to deliver more relevant and accurate search results. By understanding the underlying meaning and context of search queries, semantic search engines can surface content that is truly aligned with the user's intent, reducing the need for extensive sifting through irrelevant information.
This enhanced relevance not only improves the overall user experience but also increases the chances of users finding the information they need, potentially leading to higher engagement, conversions, and customer satisfaction.
Enhanced Personalization
Semantic search leverages contextual factors, such as user history, location, and device, to personalize the search experience. By tailoring the results to the individual user's preferences and needs, semantic search engines can provide a more tailored and valuable experience, further increasing user satisfaction and engagement.
This personalization aspect is particularly crucial in today's digital landscape, where users expect customized experiences that cater to their unique preferences and requirements.
Increased Discoverability
Semantic search can dramatically improve the discoverability of content, products, and services. By understanding the broader context and relationships between search queries and content, semantic search engines can surface relevant results that may have been difficult to find using traditional keyword-based search.
This enhanced discoverability can be especially beneficial for businesses, as it can help them reach a wider audience and increase the visibility of their offerings, leading to potential increases in website traffic, leads, and conversions.
Deeper Insights and Informed Decision-Making
The integration of semantic search capabilities can provide businesses with valuable insights that can inform strategic decision-making. By analyzing the patterns and trends in user search queries, businesses can better understand their customers' needs, interests, and pain points, enabling them to develop more targeted and effective marketing and product strategies.
Furthermore, the ability to extract and interpret the contextual meaning behind search queries can help businesses identify emerging trends, untapped opportunities, and potential areas of growth, ultimately giving them a competitive edge in their respective industries.
Improved User Experience
At the heart of semantic search lies the goal of enhancing the overall user experience. By delivering more accurate, relevant, and personalized search results, semantic search engines can reduce the time and effort required for users to find the information they need, ultimately improving their satisfaction and engagement with the search platform.
This improved user experience can have a ripple effect, leading to increased brand loyalty, higher customer retention, and greater overall success for businesses that effectively leverage semantic search capabilities.
Implementing Semantic Search: Strategies and Best Practices
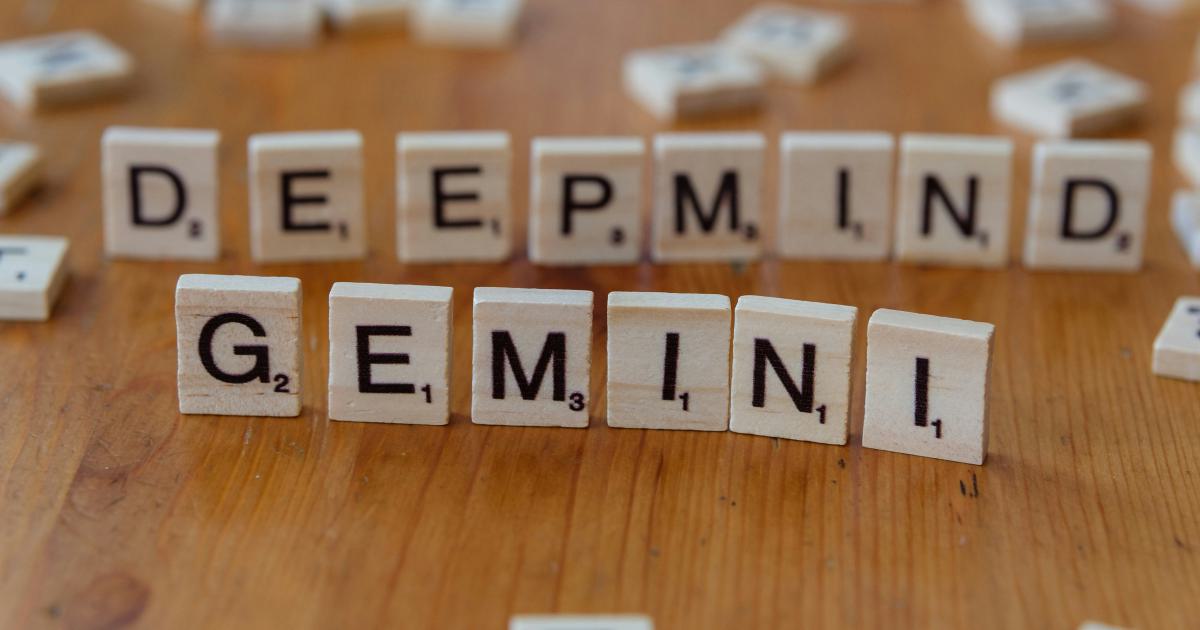
As businesses and organizations strive to harness the power of semantic search, it is crucial to understand the key strategies and best practices for successful implementation.
Optimize Content for Semantic Search
One of the foundational steps in leveraging semantic search is ensuring that the content on your website or platform is optimized for semantic search engines. This involves:
Enhancing Content Quality: Focus on creating high-quality, comprehensive, and informative content that addresses the user's needs and intent, rather than simply stuffing keywords.
Incorporating Semantic Markup: Utilize structured data and schema.org markup to help search engines better understand the entities, relationships, and context within your content.
Optimizing for Natural Language: Optimize your content to cater to natural language queries, using conversational language and addressing common questions and pain points.
Leveraging Synonyms and Related Terms: Incorporate synonyms, related concepts, and topical variations within your content to improve semantic understanding.
Leverage Contextual Signals
Semantic search engines rely heavily on contextual signals to understand the user's intent and provide relevant results. Businesses should focus on collecting and integrating the following types of contextual data:
User Behavior and Preferences: Track user search history, browsing patterns, and other behavioral data to personalize the search experience.
Location and Device Information: Utilize location and device data to deliver location-specific and device-optimized search results.
Social and Engagement Signals: Incorporate social media interactions, shares, and other engagement metrics to better understand content relevance and popularity.
Continuously Refine and Improve
Implementing semantic search is an iterative process that requires ongoing refinement and improvement. Businesses should regularly monitor the performance of their semantic search implementation, analyze user feedback and search data, and make adjustments to their strategies and content to optimize for better results.
This may involve:
Analyzing Search Queries and Intent: Closely study user search queries and their underlying intent to identify areas for improvement and optimization.
Testing and Experimenting: Conduct A/B testing and experiments to evaluate the effectiveness of different semantic search approaches and content strategies.
Staying Informed on Industry Trends: Stay up-to-date with the latest advancements in semantic search technology and best practices to ensure your implementation remains cutting-edge.
Collaborate with Subject Matter Experts
Successful implementation of semantic search often requires the expertise of cross-functional teams, including:
Content Strategists: Work with content strategists to ensure that your content is optimized for semantic search and addresses user intent.
Data Analysts: Collaborate with data analysts to extract and interpret the insights from user search data and contextual signals.
Technical Experts: Engage with technical experts, such as developers and engineers, to integrate semantic search capabilities seamlessly into your digital platforms.
By leveraging the collective knowledge and expertise of these subject matter experts, businesses can create a comprehensive and effective semantic search strategy that delivers tangible results.
The Future of Semantic Search
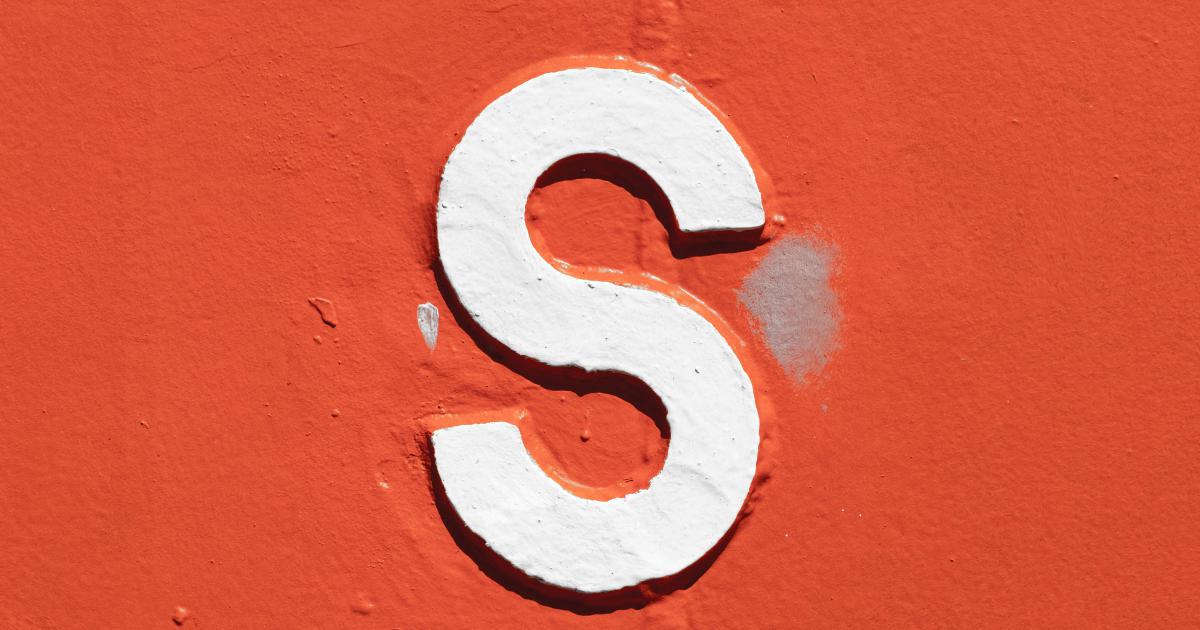
As the digital landscape continues to evolve, the role of semantic search in shaping the future of information discovery and access is becoming increasingly prominent. Here are some of the key trends and developments that are likely to shape the future of semantic search:
Advancements in Natural Language Processing (NLP)
The continued advancements in natural language processing (NLP) will play a pivotal role in the future of semantic search. As NLP algorithms become more sophisticated in understanding the nuances of human language, search engines will be able to provide even more accurate and contextual results, catering to the growing demand for natural, conversational search experiences.
Increased Adoption of Voice Search and Virtual Assistants
The rise of voice search and virtual assistants, such as Alexa, Siri, and Google Assistant, has already driven a significant shift towards more natural, conversational search queries. As these technologies become more prevalent, the need for robust semantic search capabilities will only increase, as search engines must be able to comprehend and respond to these more complex, context-driven requests.
Integration of Artificial Intelligence and Machine Learning
The fusion of semantic search with advanced artificial intelligence (AI) and machine learning (ML) algorithms will further enhance the precision and personalization of search results. By leveraging AI-powered techniques, such as deep learning and neural networks, search engines will be able to better understand user intent, anticipate their needs, and provide even more tailored and relevant information.
Expansion of Knowledge Graphs and Structured Data
The continued growth and refinement of knowledge graphs and structured data will play a crucial role in the future of semantic search. As search engines have access to more comprehensive and interconnected datasets, they will be able to uncover deeper insights, make more informed inferences, and provide users with a richer, more contextual search experience.
Increased Focus on User Experience and Personalization
As user expectations and demands continue to evolve, the future of semantic search will place a greater emphasis on delivering personalized, intuitive, and seamless user experiences. Search engines will need to prioritize the integration of user preferences, behavioral patterns, and contextual signals to provide truly tailored and relevant search results, further enhancing user satisfaction and engagement.
Emergence of Domain-Specific Semantic Search
While the current focus of semantic search has been primarily on general web search, the future may see the rise of specialized, domain-specific semantic search solutions. These niche search engines will leverage deep subject matter expertise and tailored knowledge graphs to deliver highly targeted and accurate results within specific industries or areas of interest, catering to the unique needs of specialized user communities.
As the digital landscape continues to expand and evolve, the role of semantic search in transforming the way we access and consume information will only become more crucial. By embracing these emerging trends and innovations, businesses and individuals can stay ahead of the curve and harness the full potential of semantic search to unlock new opportunities for discovery, knowledge-sharing, and strategic decision-making.